In the last piece in this series on developing with Flutter, we looked at how we can implement [image labeling using ML Kit, which belongs to the Firebase family.
In this 7th installment of the series, we’ll keep working with ML Kit, this time focusing on implementing face detection. The application we build will be able to detect human faces in an image, like so:
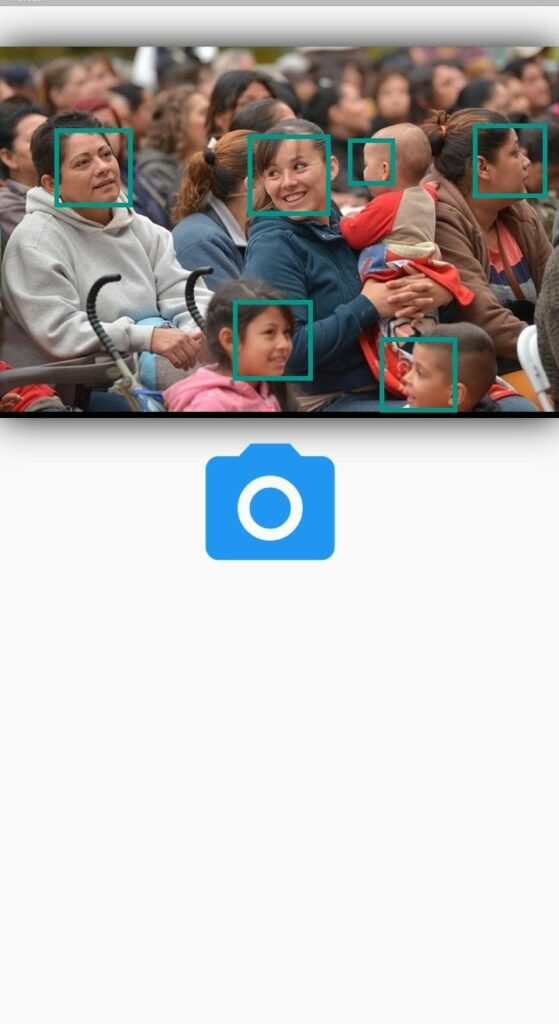
Application and Use Cases
Using face detection in Firebase’s ML Kit enables you to detect faces in an image, without providing additional data. The face detection algorithm returns rectangular bounding boxes that you can then plot on the detected faces. It’s also able to detect key facial landmarks such as eyes, mouth, nose, etc. Some of its applications include generating avatars from images and adding filters to images.
Add Firebase to Flutter
Just like in the previous piece, the first step involves adding Firebase to your Flutter project. This is done by creating a Firebase project and registering your app. Follow the comprehensive guide below to set that up:
Install Required Packages
Now that your app’s communication with Firebase is set up, you have to install two packages. The first is image_picker and the second is firebase_ml_vision. We’ll use the image_picker to pick the images from the phone’s gallery or to take a new picture.
firebase_ml_vision will provide the face detection functionality.
Select an Image
At this point, we can now select the image we’d like to detect faces in. We can choose an image either from the gallery or the camera. We start by declaring a couple of variables:
- pickedImage will hold the image selected from the gallery or camera, of type File
- imageFile will contain the same image, of type image
- rect will contain the rectangular positions of the bounding boxes
- isFaceDetected will signal whether or not face(s) have been detected
Next, we declare an asynchronous function called pickImage that holds all the functionality. The function returns a Future because some of its aspects, such as loading an image, return a Future.
We then use the ImagePicker package to pick the image. In order to use a camera image, change the source to ImageSource.camera. At some point, we’ll need to paint the image on the screen. Therefore, we have to convert the loaded awaitImage to be of type Image. We do this by applying the decodeImageFromList() function to the imageFile after reading it as bytes.
Finally, we set the images in the state function:
Create a FirebaseVisionImage
In this step, we create a FirebaseVisionImage object from the selected image:
Create an Instance of a Detector
The next step is to create an instance of the detector that we’d like to use. Here, we’re using the face detector:
Process the Image
We’re now ready to process the image using the detector.
Extract the Faces
At this point, we can now start extracting faces. However, we notice that after running the application once, the bounding boxes of the previously detected faces remain on the screen. A visual of the problem is shown below.
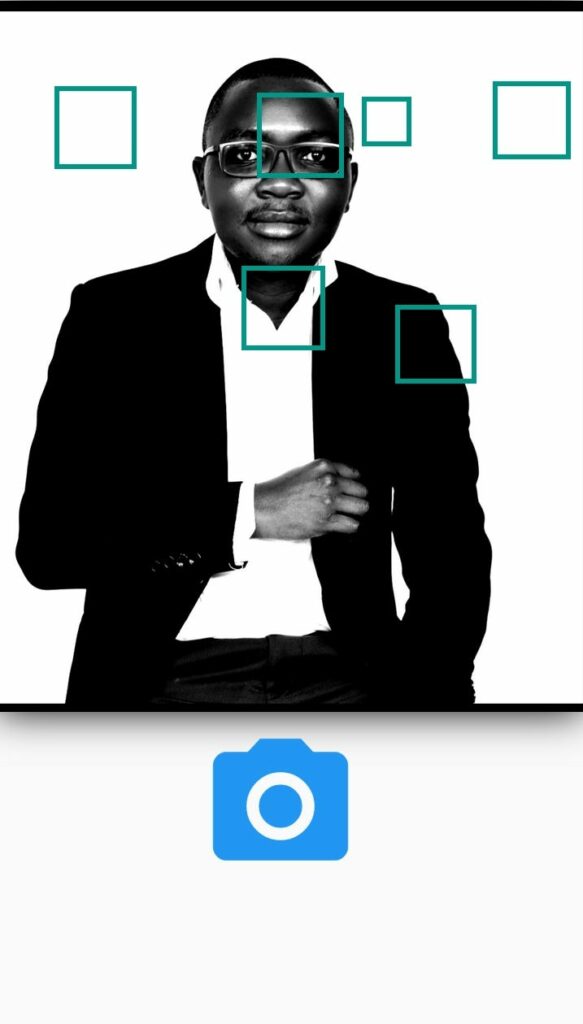
In order to solve this problem, we clear all the rectangular bounding boxes whenever a new image is selected.
At this point, we can loop through all the detected faced and add their bounding boxes to the rect. We can also obtain the x and y rotations of the head.
Here’s a visual of the obtained rotations.
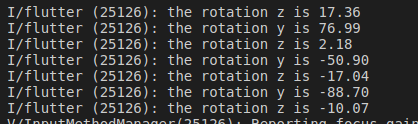
A visual of the bounding box rectangles obtained is shown below.
The only task remaining now is to paint these bounding boxes on top of the faces that were detected.
Draw the Bounding Boxes
In order to draw the bounding boxes accurately, we need to ensure that the image is in a fitted box. If we were to display the image with its default size, it would be difficult to display the bounding boxes, because large images would go outside the device’s area of view.
FacePainter is the class that will display the image together with the bounding boxes. The CustomPaint widget allows us to paint objects on the screen.
In order to pain objects on the screen, we create a custom FacePainter class that extends the CustomPainter . We loop through all the rectangles as we draw the bounding boxes on the screen. Setting shouldRepaint to true ensures that the bounding boxes are repainted when we select a new image and new bounding boxes become available.
class FacePainter extends CustomPainter {
List<Rect> rect;
var imageFile;
FacePainter({@required this.rect, @required this.imageFile});
@override
void paint(Canvas canvas, Size size) {
if (imageFile != null) {
canvas.drawImage(imageFile, Offset.zero, Paint());
}
for (Rect rectangle in rect) {
canvas.drawRect(
rectangle,
Paint()
..color = Colors.teal
..strokeWidth = 6.0
..style = PaintingStyle.stroke,
);
}
}
@override
bool shouldRepaint(CustomPainter oldDelegate) {
return true;
}
}
In this article, we covered the major building blocks for this application. The entire source code for this application can be viewed from the link below:
Final Thoughts
You can clearly see how fast and easy it is to incorporate machine learning in your mobile apps using Firebase’s ML Kit. Before you can launch this application into production, ensure that you check with Firebase’s checklist to make sure you have the right configurations.
Should you choose to use the cloud-based API, ensure that you have the right API access rights. This will ensure that your application doesn’t fail in production.
Using the repo below, you can try out different detectors.
Comments 0 Responses