If you’re even remotely plugged into the tech world, you’d have been hard-pressed to miss a couple viral summer trends, both involving AI-powered photo transformations.
Here’s the gist. Snapchat caught fire and soared past all Q2 estimates, in large part because of their rollout out of popular gender-swap and baby-face Lenses. And soon after, FaceApp took the internet by storm when its old-age filter went viral. This has led to millions of users, including prominent celebrities, showing off what they’d look like in 40 years or as members of a different gender.
On the surface, this is an app developer’s dream. Viral usage like this can significantly boost key metrics like daily active users (DAUs), downloads, engagement, revenue, and more. But despite the foundational similarities between the two companies’ ML-powered filters, the public response and bottom line impacts couldn’t have been more different.
Though there are other factors at play (political, brand awareness, market share, etc.), we can view Snapchat’s boom and FaceApp’s bust through the lens of how each company handles user data.
The promise of augmented reality and machine learning on mobile is real. But in order to avoid PR nightmares, developers and tech execs both need to be aware of the data privacy issues underlying these tools.
Snapchat’s Viral Moment
Snapchat’s 2019 Q2 report makes clear the potential benefits of this kind of virality:
- DAUs reached 203M in Q2 2019, compared to 190M in Q1 2019 and 188M in Q2 2018. This growth comes on the heels of several quarters with stagnant and even slightly decreasing DAU.
- This 13M increase in DAU was the highest total in 3 years for Snapchat, with an estimated 7–9M of those new DAUs coming as a result of the Lenses, according to CFO Derek Andersen.
- By the end of July, Snap’s share price had surpassed its $17/share IPO, following two years of struggles as a public company. If even half of Snap’s market cap increase (+$4.21B) from Q1-Q2 can be attributed to the new Lenses, that would account for more than $2B in value added to the company.
- More than 200M Snapchatters used the new filters within the first two weeks of their release.
- Reported revenue was $388M vs $360.5M expected.
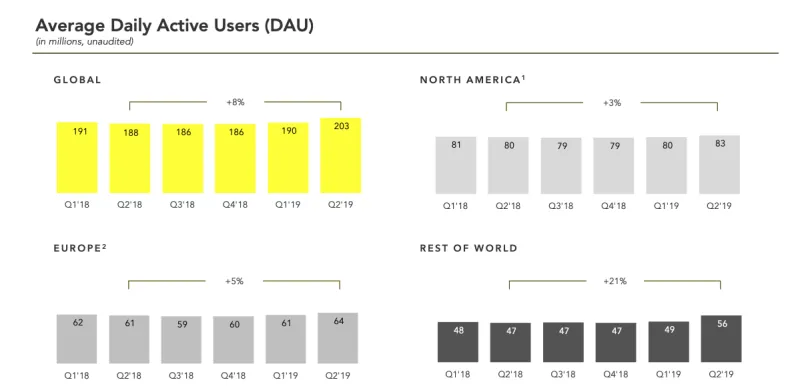
While Snapchat’s AI-powered Lenses might not account for all of these gains, it’s fair to assume that their viral momentum over the summer played a major role in these impressive quarterly numbers.
FaceApp’s Viral Moment
We also saw the “FaceApp Challenge” sweep across social media — for a number of days, you couldn’t browse Twitter or Instagram for more than a minute before coming across decades-aged selfies from everyday users and celebrities alike.
But if you’re expecting another roundup of improved metrics, you’ll be surprised to hear the vastly different response FaceApp garnered in light of its viral success.
From tech outlets warning of aggressive data collection to the U.S. Democratic National Committee urging its candidates to not use the app over security concerns, the controversy surrounding FaceApp and data privacy has been swift and wide-ranging.
While the gravest concerns have been dispelled and the political dangers (somewhat) quelled, FaceApp is still struggling with a highly negative public relations moment and has had to proactively respond to these questions about user privacy.
Data privacy and on-device machine learning
When considering these two viral moments, a question arises: If Snapchat and FaceApp are both powering these mobile experiences with the same fundamental technology (machine learning), then why the discrepancy between Snapchat’s success and FaceApp’s crisis?
As I mentioned, there are, of course, possibilities that extend beyond the scope of the underlying technology. But one key and obvious difference lies in how the tech is delivered to users.
For years now, Snapchat has been at the forefront of on-device machine learning, wherein a ML model is stored on the device (i.e. a user’s smartphone), right where data (i.e. a selfie) is being captured. As such, this data is processed locally, and users get to choose who they share their data with — their friends, a wider range of users, or Snapchat itself. This kind of user data control drastically limits exposure to hackers, 3rd parties, and the data’s perpetual existence in the cloud.
FaceApp, on the other hand, processes each image a user uploads on a cloud-hosted server and then sends the AI-edited photo back down to the device. But as noted by Thomas Brewster in Forbes, FaceApp “could operate differently. It could, for instance, process the images on your device, rather than take submitted photos to an outside server.” This reliance on cloud-based ML brings into play all the risks I mentioned above, in addition to the threats posed by a costly PR crisis.
The Takeaway
As we speed into an era of increasingly personalized and technically-impressive mobile apps, the need to secure user data will become essential.
In this particular case, we have viral moments involving two high-performing and highly-similar mobile experiences, both with machine learning at their core.
One resulted in quarterly metrics that surpassed all estimates by sizable margins; the other in a public relations nightmare that also led to a groundswell of cultural debate about data privacy and the risks of cloud-based data processing.
The ramifications are clear. Adding machine learning features to mobile apps creates the very real potential for accelerated growth. Users gravitate towards these kinds of experiences, but they also want and deserve to know what’s happening with the data they’re offering up.
To ensure this kind of data privacy while still providing cutting-edge experiences that delight their users, app developers will need to embed machine learning models on-device. If they do, we should see more breakthroughs like Snapchat’s, and fewer PR disasters like FaceApp’s.
Comments 0 Responses