Introduction
Research in computer vision has been booming over the past few years, thanks to advances in deep learning, increases in computing storage, and the explosion of big visual datasets. Every day, there are more computer vision applications in fields as diverse as autonomous vehicles, healthcare, retail, energy, linguistics, and more.
In this article, I’ll present the 5 major trends that have dominated computer vision research in 2018. An exhaustive review is impossible, so I’ll only share some of the accomplishments in the field that have most impressed me.
1 — Synthetic Data
Synthetic data has been a huge trend in computer vision research this past year. They are data generated artificially to train deep learning models. For example, the SUNCG dataset is used for simulated indoor environments, the Cityscapes dataset is used for driving and navigation, and the SURREAL dataset of synthetic humans is used to learn pose estimation and tracking. Let’s look at some of the best work utilizing synthetic data this year:
- In How Well Should You Label, the authors look at how coarsely the training labels must be to produce good segmentation quality from modern CNN architectures. This is important because synthetic data are usually known for their pixel-perfect quality. Performing their experiments on the Auto City dataset, the authors prove that the final segmentation quality is indeed strongly correlated with the amount of time spent labeling, but not so much with the quality of each individual label.
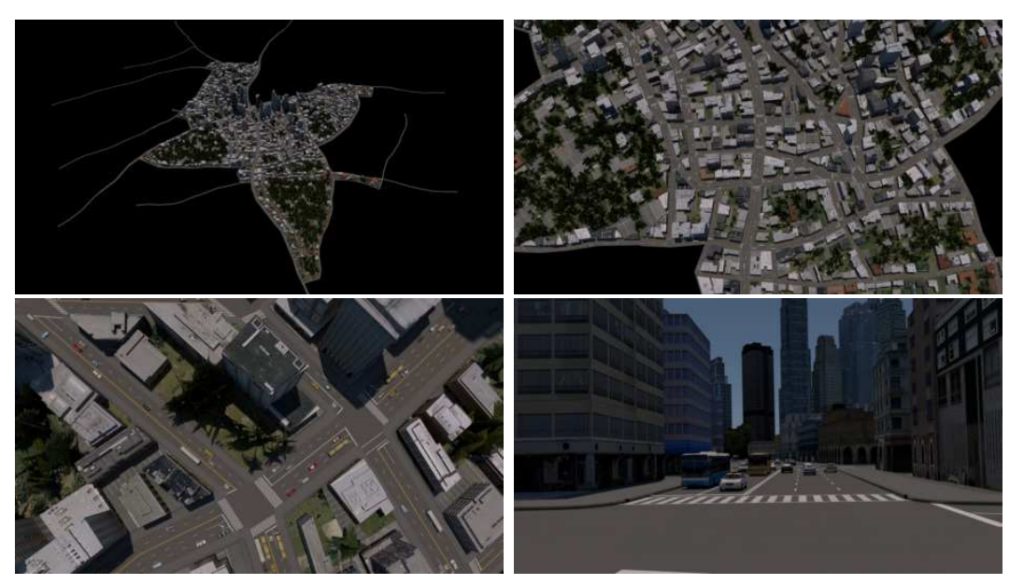
- Soccer on Your Tabletop presents a system that can take a video stream of a soccer game and transform it into a moving 3D reconstruction that can be projected onto a tabletop and viewed with augmented reality. The system extracts bounding boxes of the players, analyzes the human figures with pose and depth estimation models, and finally produces an astoundingly accurate 3D scene reconstruction.
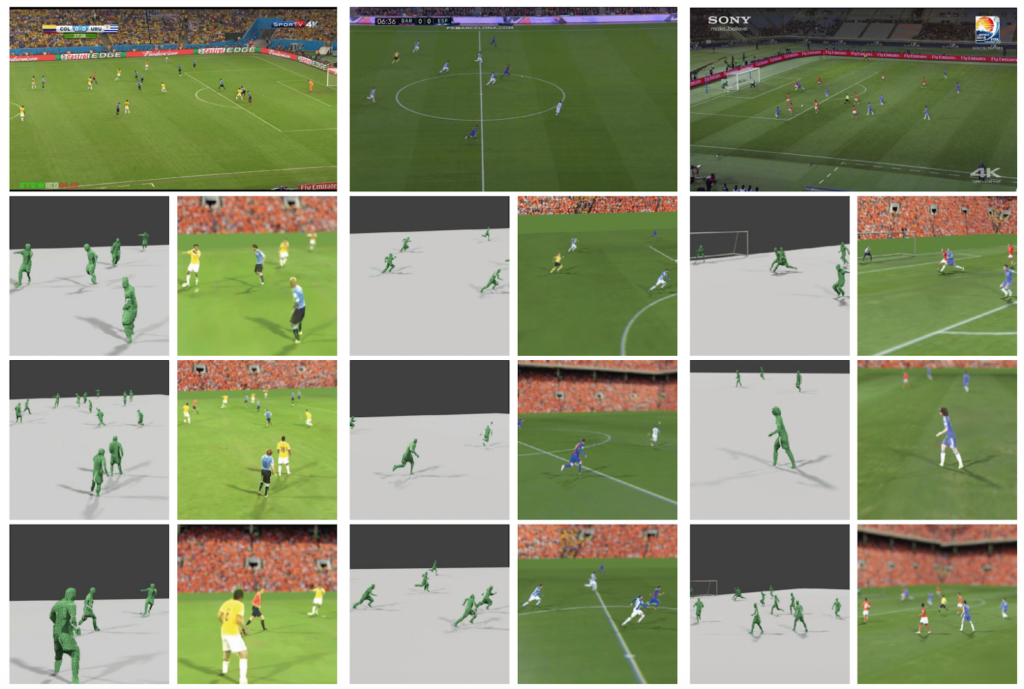
- The human ability to simultaneously learn from various information sources is still lacking in most existing feature learning approaches. Cross-Domain Self-supervised Multi-task Feature Learning using synthetic imagery addresses this gap by proposing an original multi-task deep learning network that uses synthetic imagery to better learn visual representations in a cross-modal setting. Training the network through synthetic images dramatically reduces data annotations needed for multitask learning, which is costly and time-consuming. To bridge the cross-domain gap between real and synthetic data, adversarial learning is employed in an unsupervised feature-level domain adaptation method, which enhances performance upon the transfer of acquired visual feature knowledge to real-world tasks.
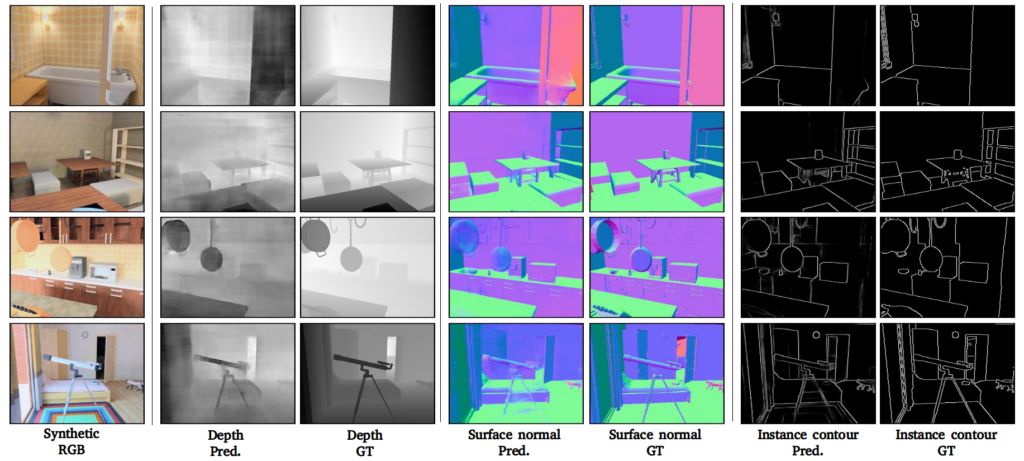
- Training Deep Networks with Synthetic Data proposes a refined approach for training deep neural network data for real object detection, relying on domain randomization of synthetic data. Domain randomization reduces the need for high-quality simulated datasets by intentionally and randomly disturbing the environment’s textures to force the network to focus and identify the main features of the object. To augment the process’s performance, additional training on real data in conjunction with synthetic data is performed, which bridges the reality gap, therefore yielding better performance results. Different approaches were proposed to exploit the potential of synthetic data, which suggests this area will further advance in the coming years.
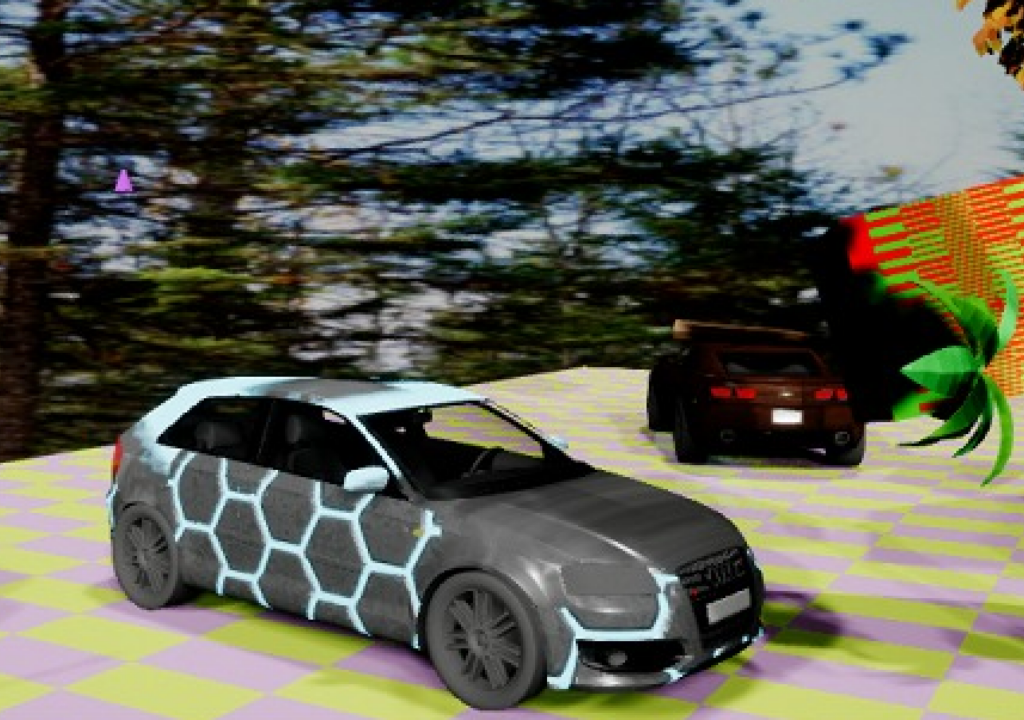
2 — Visual Question Answering
Visual question answering (VQA) is a new and exciting problem that combines NLP and computer vision techniques. It typically involves showing an image to a computer and asking a question about that image that the computer must answer. The answer could be in any of the following forms: a word, a phrase, a Yes/No answer, multiple choice answers, or a fill-in-the-blank answer.
There have been various datasets developed recently to tackle this task, such as DAQUAR, Visual7W, COCO-QA, VQA. Let’s look at some of the best models in question answering this year.
- Embodied QA reaches toward the goal of creating fully intelligent agents that can actively perceive, naturally communicate in an environment-grounded dialogue, and act and execute commands. Through goal-driven intelligent navigation of a 3D setting, the agent is asked to answer questions based on object recognition and visual grounding and understanding. Interestingly, the agent solely uses egocentric vision to navigate its surroundings. This means that the agent is not provided with a map and trained only via raw sensory inputs (pixels and words) and must rely on common sense in navigating an unfamiliar environment.
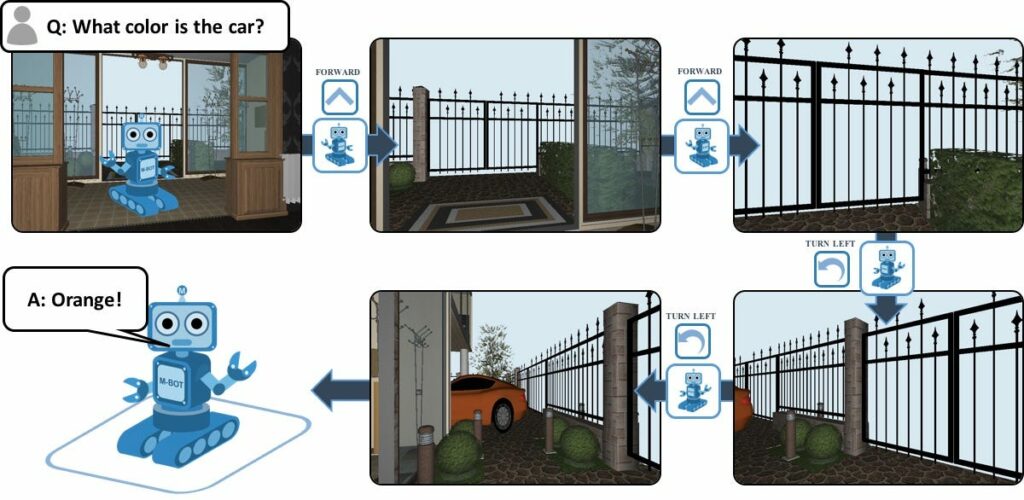
- Standard VQA models passively rely on large static datasets—unlike the interactive nature of human learning that’s more sample efficient and less redundant. Learning by asking questions fills this research gap by introducing a more interactive VQA model that mimics natural learning. In this paper, the agent is trained to learn like a human by evaluating its prior acquired knowledge and asking good and relevant questions that maximize the learning signal from each image-question pair sent to the oracle. The paper also shows how interactive questioning significantly reduces redundancy and the required number of training samples to achieve accuracy increases of 40%.
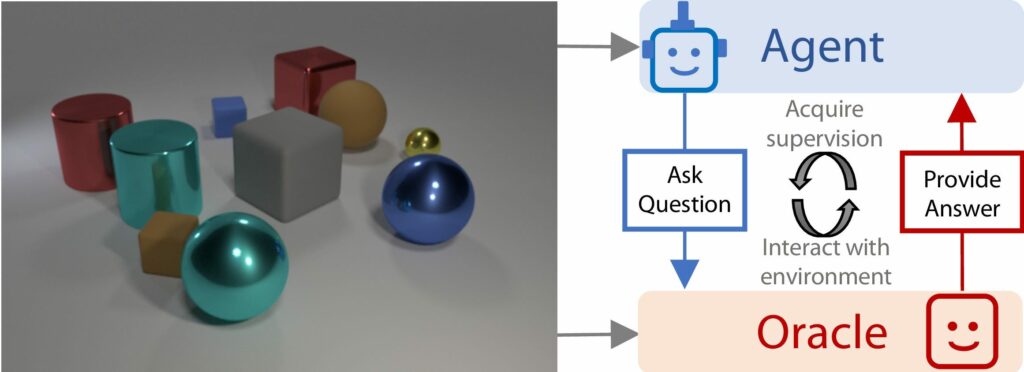
- Inverse Visual QA (iVQA) joins the other models that aim to improve the performance of standard VQA models by focusing on developing visual grounding. This paper inverses the popular VQA task so that the target is to generate a question given an image/answer pair. The learning biases of standard VQAs undermine the evaluation process. iVQA uses partially-generated questions with less biased learning priors corresponding to an image-answer pair to achieve more visual grounding.
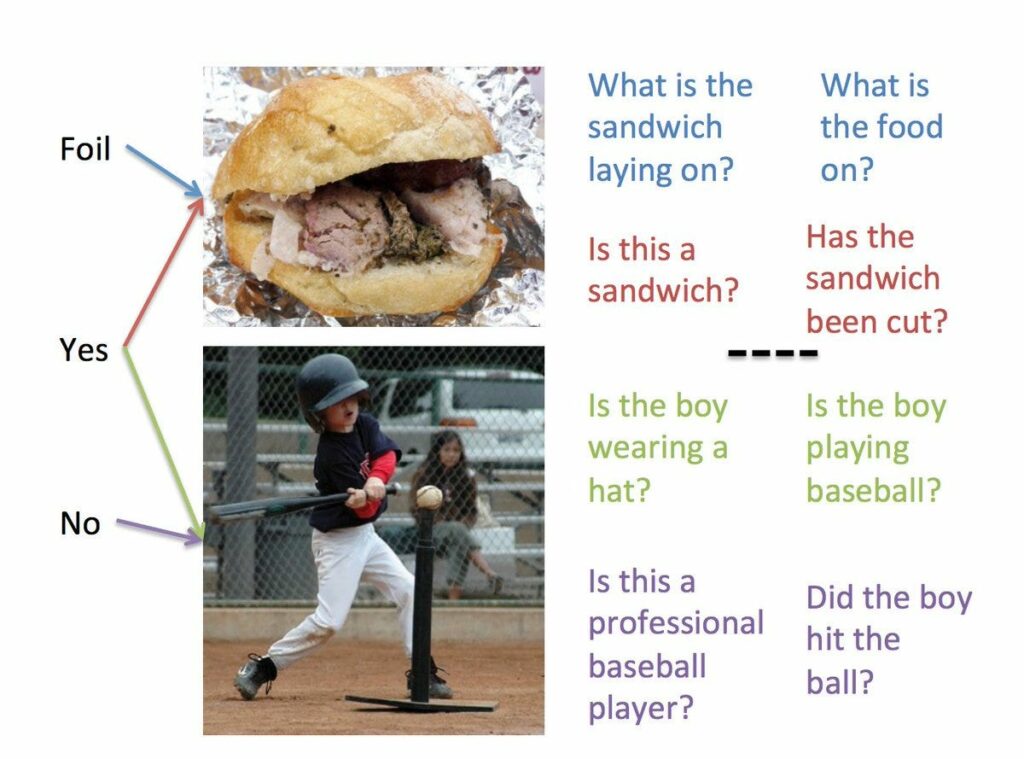
- Interactive QA addresses one of the shortcomings of standard VQA models, which are mostly passive and do not train a fully intelligent agent capable of navigating, interacting, and performing tasks within its environment. The model uses a multi-level controller method with semantic spatial memory and collects a rich dataset of simulated realistic scenes and a wide range of questions to evaluate the model. It advances standard VQA towards the ultimate goal of creating fully visually intelligent agents.
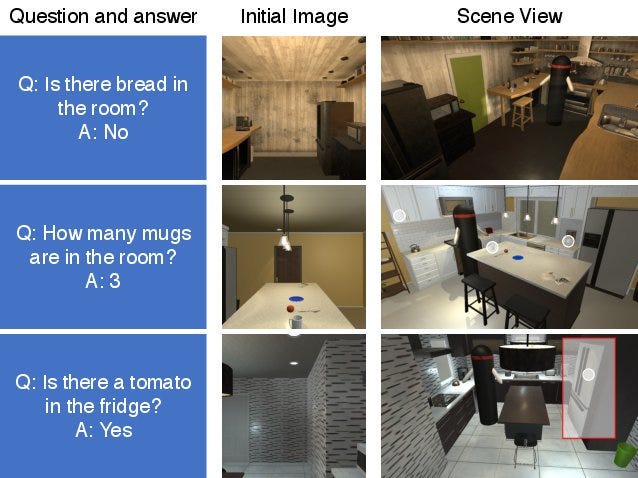
- Effectively evaluating the performance of current state-of-the-art VQA models and preventing them from relying on biased training priors is an area that is still under development. To that end, the Grounded Visual QA model offers a new method that directly dissociates the objects recognized from plausible prior answers, forcing the model to be more visually grounded. With the excellent results the paper has reported and the current community focus on this line of research, it’s a promising sign for future innovative methods further advancing VQA models.
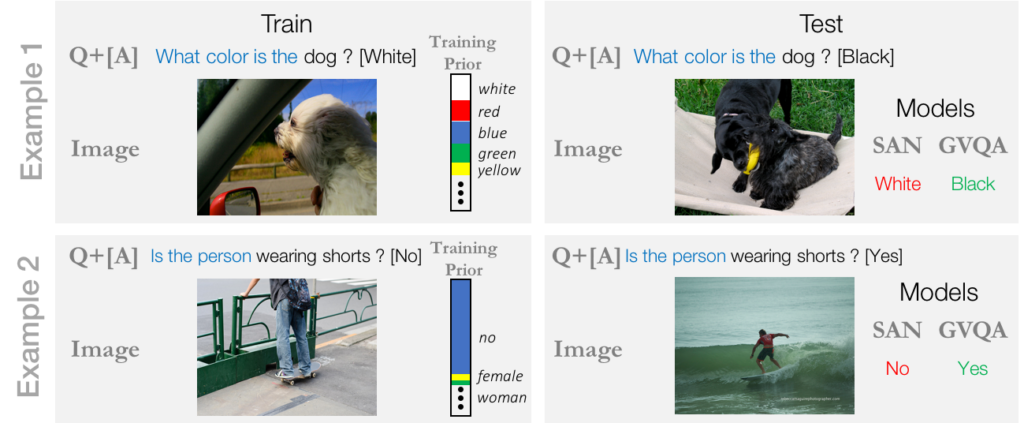
3 — Domain Adaptation
There are a couple of specific research directions that have been trending in 2018, and one is domain adaptation. This field is actually closely related to synthetic data. It tackles the big challenge of collecting labeled datasets for supervised learning and ensuring that the data are reliable and diverse enough. Essentially, how do we use one kind of data to prepare the network to cope with a different kind?
- Unsupervised Domain Adaptation with Similarity Learning deals with domain adaptation using adversarial networks. The author asks one network to extract features from a labeled source domain and another network to extract features from an unlabeled target domain, with similar but different data distribution. The classification in which the model is trained to discriminate the target prototype from all other prototypes is different. To label the image from the target domain, the author compares the embedding of an image with embeddings of prototype images from the source domain and then assigns the label of its nearest neighbors.
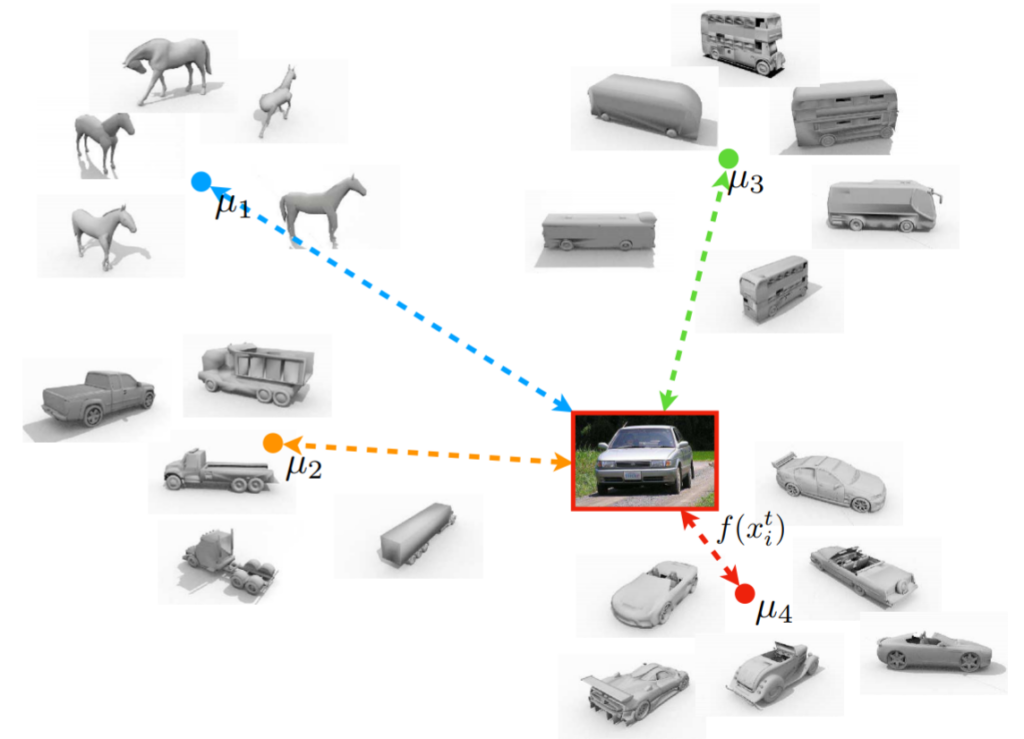
- Image to Image Translation for Domain Adaptation looks at domain adaptation for image segmentation, which is used widely in self-driving vehicles, medical imaging, and many other domains. Basically, domain adaptation techniques here must find a mapping structure from the source data distribution to the target data distribution. The approach uses 3 main techniques: (i) domain-agnostic feature extraction (the distributions of features extracted from both source and target domains are indistinguishable), (ii) domain-specific reconstruction (embeddings can be decoded back to the source and target domains), and (iii) cycle consistency (mappings are learned correctly).
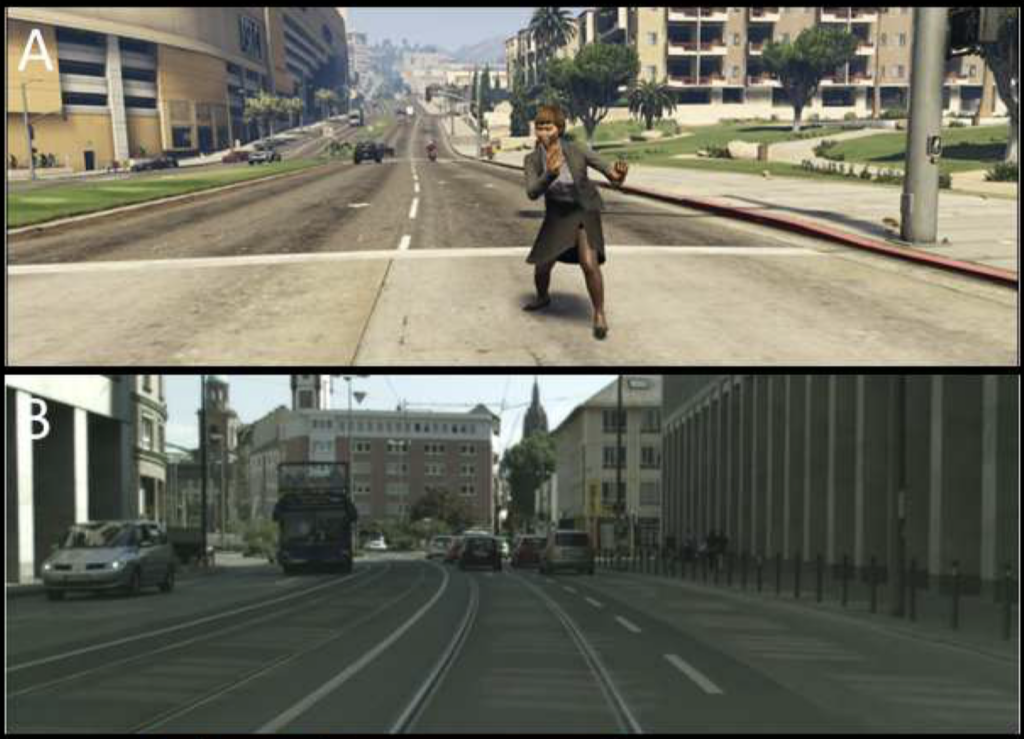
- Conditional GAN for Structured Domain Adaptation offers a new method to overcome the challenges of cross-domain differences in semantic segmentation models with a structured domain adaptation method. Unlike unsupervised domain adaptation, the method does not assume the existence of cross-domain common feature space, and rather employs a conditional generator and a discriminator. Therefore, a conditional GAN is integrated into the CNN framework that transfers features of synthetic images to real-image like features. The method results outperform previous models, highlighting the growing potential of synthetic datasets in advancing vision tasks.
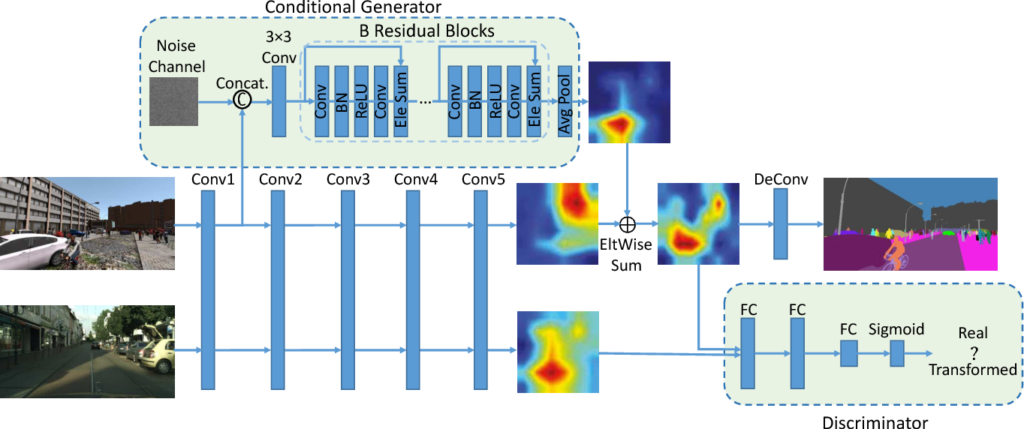
- Training deep learning-based models relies on large annotated datasets, which requires lots of resources. Despite achieving state-of-the-art performance in many visual recognition tasks, cross-domain differences still constitute a big challenge. To transfer knowledge across domains, Maximum Classifier Discrepancy for Unsupervised Domain Adaptation uses a novel adversarial learning method for domain adaptation without a need for any labeling information from the target domain. It’s observed that minimizing the discrepancy between the probability estimates from two classifiers for samples from a target domain can produce class-discriminative features for various tasks, from classification to semantic segmentation.
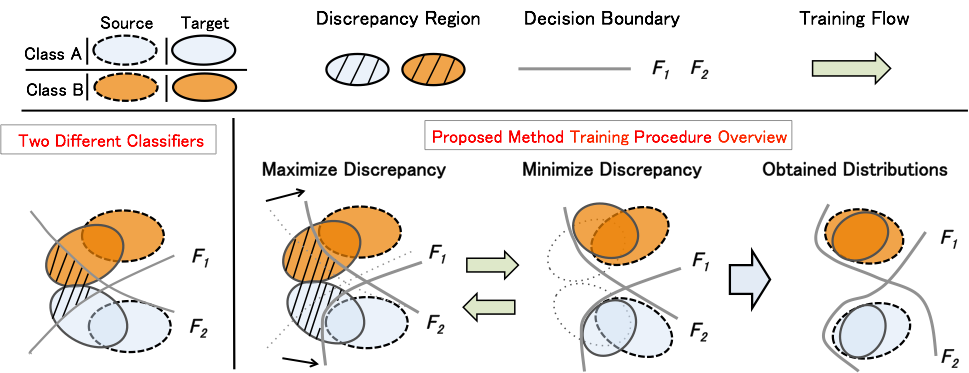
4 — Generative Adversarial Networks
2018 has definitely been a big year for Generative Adversarial Networks (GAN), the most successful class of generative models for computer vision. Let’s look at some of the best works that improve GAN models this year:
- Conditional GANs are already widely used for image modeling, but they are also very useful for style transfer. Particularly, they can learn salient features that correspond to specific image elements and then change them. In PairedCycleGAN for Makeup, the authors present a framework for makeup modification on photos. They train separate generators for different facial components and apply them separately, extracting facial components with a different network.
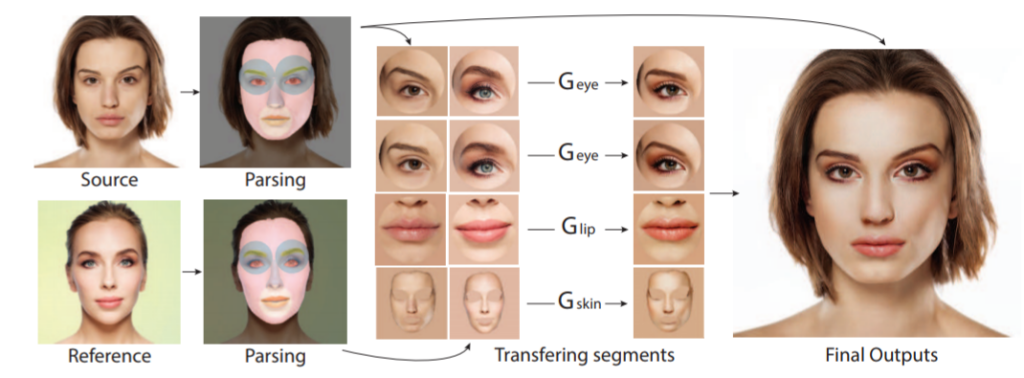
- Eye Image Synthesis with Generative Models looks at the problem of generating human eyes images. This is an interesting use case because we can use generated eyes to solve the gaze estimation problem — what is a person looking at? The authors use a probabilistic model of eye shape synthesis and a GAN architecture to generate eyes following that model.
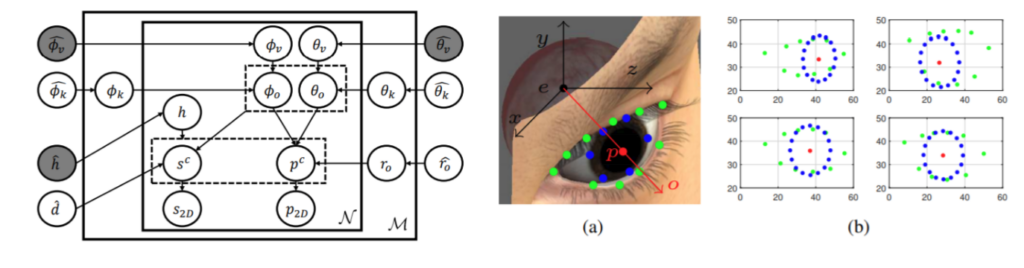
- Generative Image In-painting with Contextual Attention looks at the challenging problem of filling in blanks on an image. Usually, we need to have an understanding of the underlying scene to do in-painting. This work instead uses a GAN model that can explicitly use features from the surrounding image to improve generation.
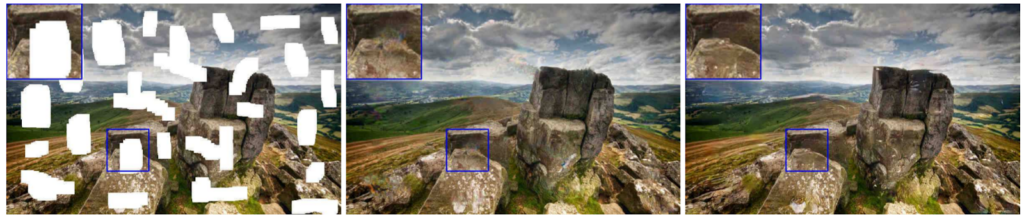
- Current state-of-the-art GAN-based text-to-image generation models encode textual descriptions only on the sentence level and overlook fine-grained information on the word-level that would improve the quality of generated images. AttnGAN proposes a novel word-level attention mechanism that’s far more impressive in producing complex scenes.
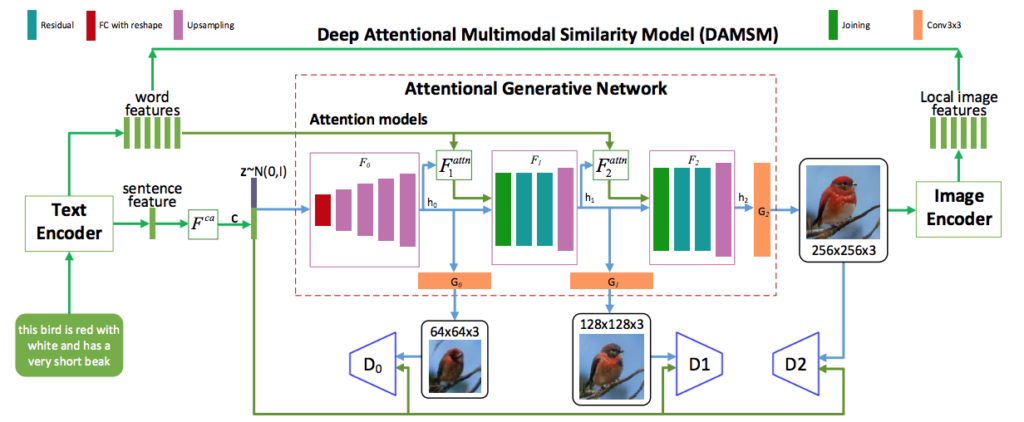
- In contrast to the common belief that the success of neural networks mainly comes from their strong ability to learn from data, Deep Image Prior demonstrates the importance of the structure of the network for building good image priors. The paper proposes a decoder network as a prior for imaging tasks. Interestingly enough, the authors show that a generator network is adequate to capture a large amount of low-level image statistics prior to any learning. The authors also use the approach to investigate the information content retained at different levels of the network by producing so-called natural pre-images. Intriguingly, using the deep image prior as a regularizer, the pre-image obtained from even very deep layers still captures a large amount of information.
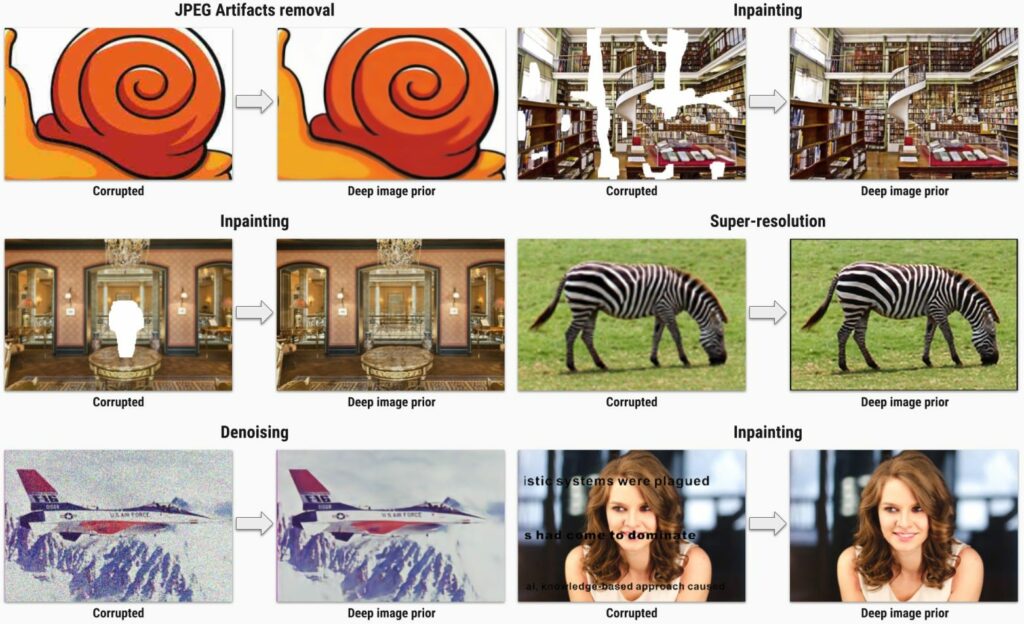
- Despite the success of GANs, no considerable success has been reported on the usage of their discriminator network as a universal loss function for common supervised tasks such as semantic segmentation. Matching Adversarial Networks highlights the reason behind this, namely that the loss function does not directly depend on the ground truth labels during generator training, which leads to random production of samples from data distributions without correlating the input-output relations in a supervised fashion. To overcome this, the paper proposes replacing the discriminator with a matching network—while taking into account both the ground truth outputs as well as the generated examples—which is facilitated by a Siamese network architecture.
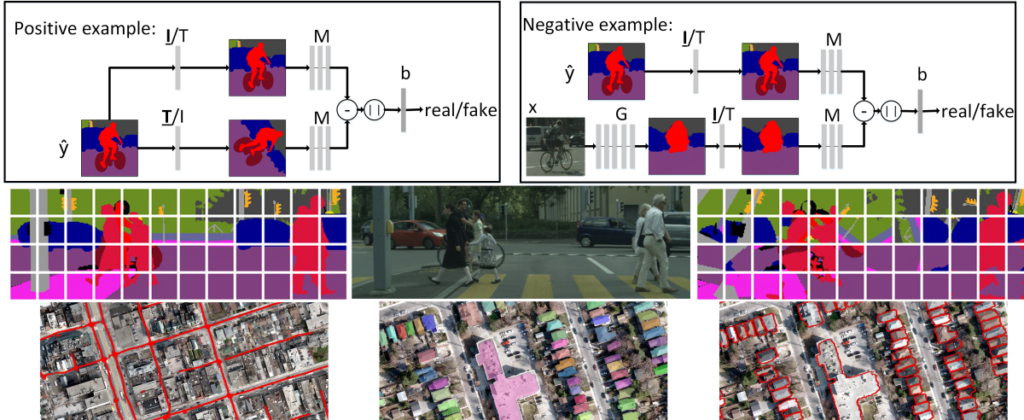
5 — 3D Object Understanding
3D object understanding is critical for deep learning systems to successfully interpret and navigate the real world. For instance, a network may be able to locate a car in a street image, color all of its pixels, and classify it as a car. But does it fully understand where the car in the image is, with respect to other objects in the street?
The work in 3D object understanding spans a wide variety of research areas including object detection, object tracking, pose estimation, depth estimation, scene reconstruction, and more. Let’s cover major papers in this field in 2018:
- Detect-and-Track is an extension of Mask R-CNN, one of the most promising approaches to image segmentation that appeared back in 2017. The authors propose a 3D Mask R-CNN architecture that uses spatiotemporal convolutions to extract features and recognize poses directly on short clips. The complete architecture can be seen below. It achieves state-of-the-art results in pose estimation and human tracking.
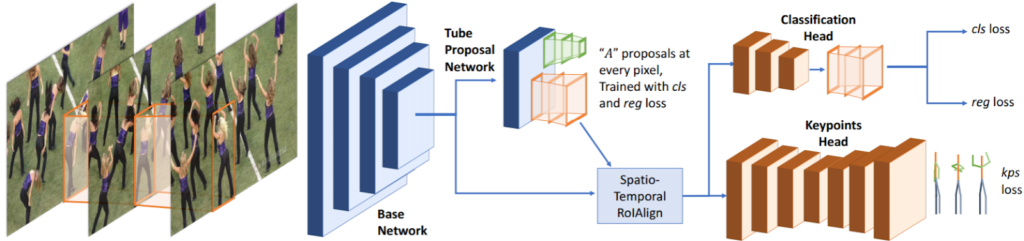
- Pose-Sensitive Embeddings for Person Re-Identification tackles the challenge of person re-identification. Usually, this problem is solved with retrieval-based methods that derive proximity measures between the query image and stored images from some embedding space. The paper instead proposes a novel way to incorporate information about the pose directly into the embedding and improve re-identification results. You can see the architecture below.
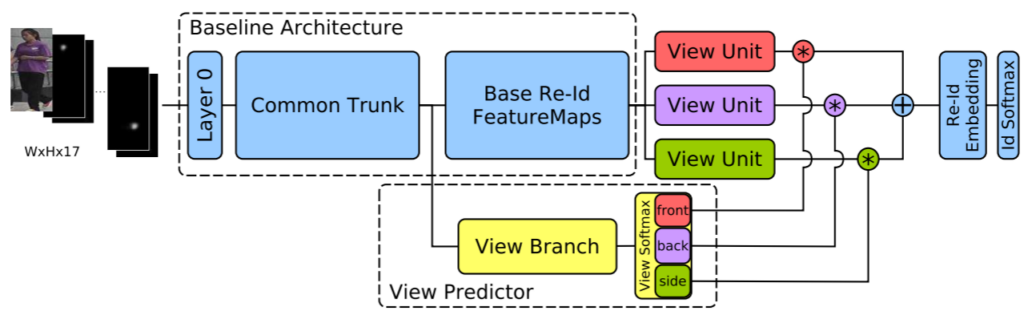
- 3D Poses from a Single Image presents a very surprising approach to pose estimation. It generates the 3D mesh of a human body directly through an end-to-end convolutional architecture that combines pose estimation, segmentation of human silhouettes, and mesh generation. The key insight is that it uses SMPL, a statistical body shape model that provides a good prior for the human body’s shape. Consequently, it manages to construct a 3D mesh of a human body from a single color image.
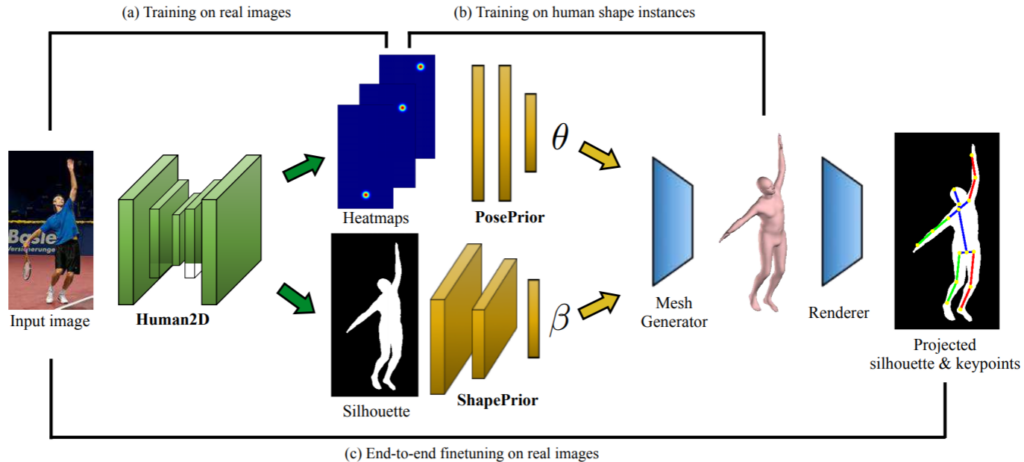
- Flow Track deals with the problem of object tracking. It’s an extension of discriminative correlation filters, which learn a filter that corresponds to the object and apply it to all video frames. The model architecture has a spatial-temporal attention mechanism that attends across different time frames in the video.
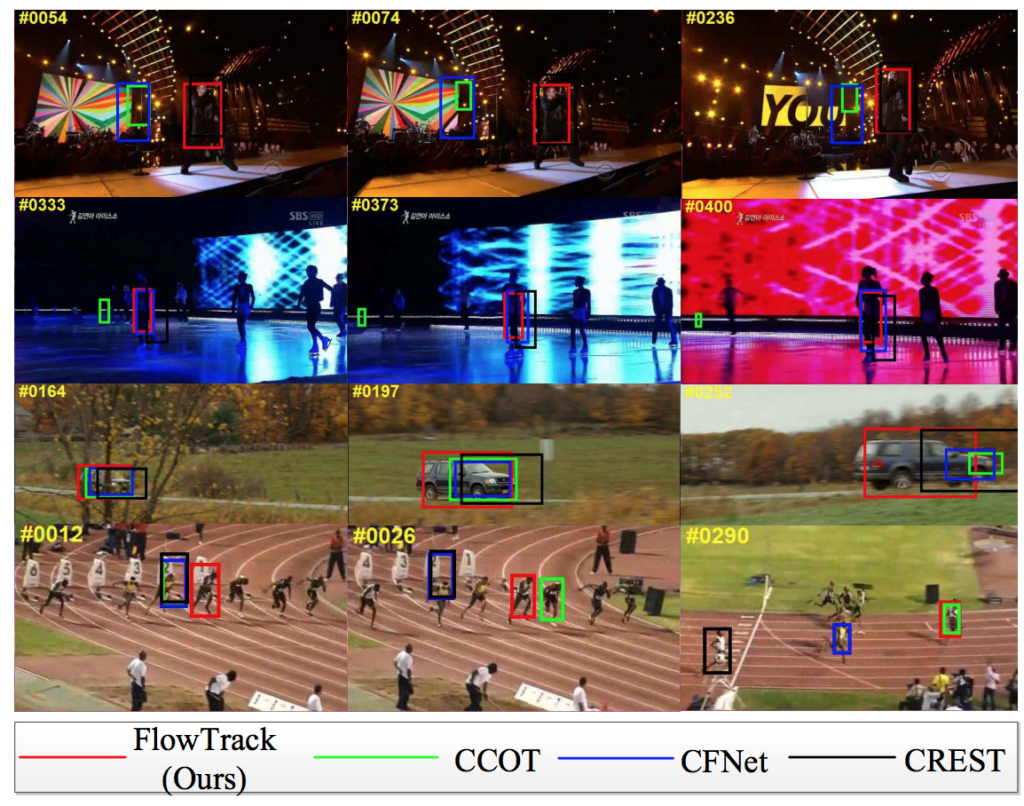
- Just like Flow Track described above, Correlation Tracking also deals with object tracking and also uses correlation filters. However, it doesn’t use a deep neural network; instead, it has reliability information — meaning that the authors add a term to the objective function that models how reliable the learned filter is.
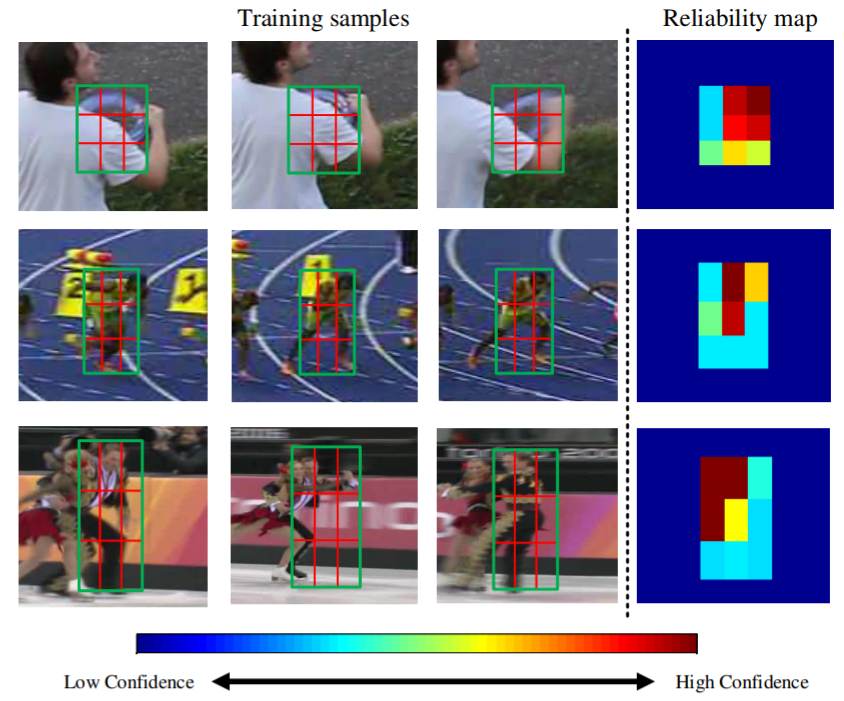
Conclusion
I hope you enjoyed reading this brief review. If you’re interested in exploring these computer vision research topics in even more detail, I’d suggest you read up on the papers presented at CVPR, ICLR, and NeurIPS — the most prestigious conferences in the field of machine learning and artificial intelligence.
Comments 0 Responses